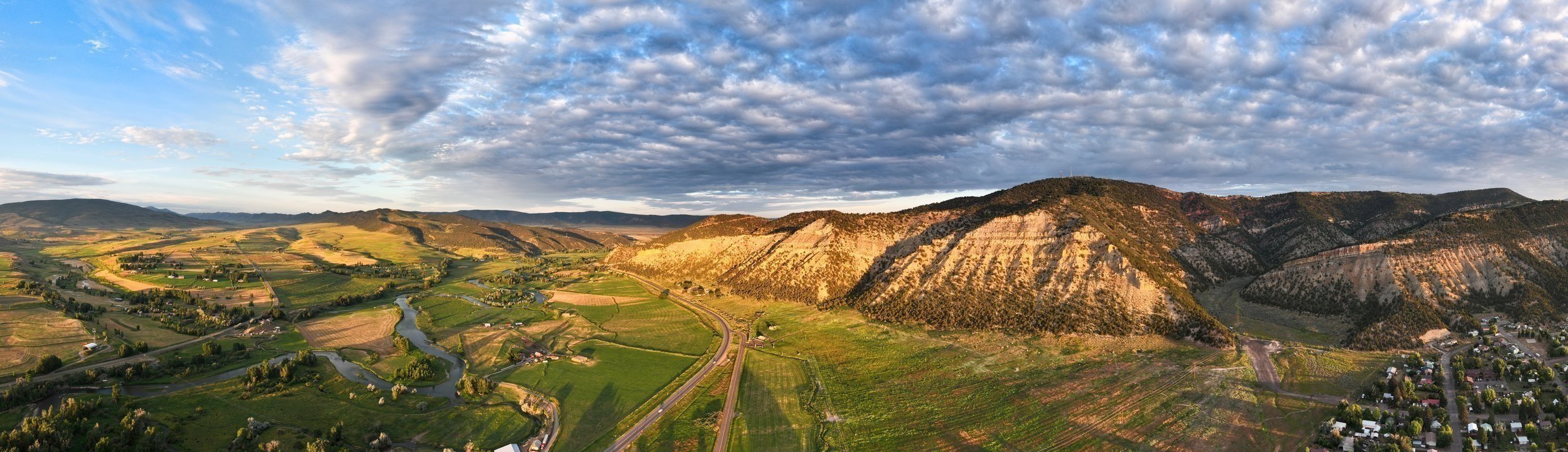
Data Analysis & Visualization
2022
In my most recent article co-authored with Dr. Tony Smith, Virtual Solar Storage Benefits All Ratepayers and Utilities, we demonstrate how solar can exhibit demand reduction properties similar to that of battery storage when applied in optimal circumstances. As a foundation of this research, I conducted in-depth analyses into the historical performance of Secure Futures’ existing solar arrays, demonstrating that solar is able to reliably and predictably reduce peak demand, both on individual customers’ bills and for the grid as a whole.
Selected results from my cover article in the Summer 2022 issue of Solar Today
This figure represents the core of our research as a highly granular look at the interface between solar generation and demand on the grid. It visualizes peak demand reduction by time of day at a cold storage facility, recreating the highest demand value at any 15-minute interval with and without solar during 2019 and 2020. Compared to what would have been a prominently day-peaking profile without solar, relatively level peak demand was maintained throughout the day with solar.
Another important aspect of this phenomenon is the reduction that customers can see in their monthly billed peak demand. This figure shows results from eight facilities with varying levels of peak demand and array sizes. Solar contributed to summer peak-load shifting from midday to mornings and evenings, primarily during weekdays. Each point represents monthly demand peaks for all facilities analyzed, including when they occurred. Summer months (May-August) are shown above, but similar patterns were found with annual peak-load shifting.
Click figures to enlarge
The full Solar Today article with additional background and figures can be found here.
Additional examples of quantitative analysis work with Secure Solar Futures
I have developed and regularly updated dozens of rate schedule models to analyze customer billing data and projected solar production. This is a key process in planning solar projects, which is used to estimate financial and energy savings and ultimately determine feasibility for prospective solar projects. A key component of this is properly sizing solar arrays to best address each customer’s energy usage patterns (more information on right-sizing).
I combined the models used for the Virtual Solar Storage research and the previously mentioned rate schedule models to determine the historical financial savings from solar to existing customers. Upon deriving monthly energy usage and peak demand offsets from interval data, this can be translated into financial savings using each rate schedule model.
I compiled data including the location and type of coal mines, retired coal plants, regional economic presence of fossil fuel industries, and socioeconomic Census data across various geographic denominations. These were then cross-referenced to determine the eligibility of hundreds of individual properties, Census tracts, and statistical areas throughout Appalachia and the Southeastern US for additional tax incentives carved out in the Inflation Reduction Act. I summarized these findings in databases for internal use as well as an interactive map.
2021
Contributions to the February 2021 urban heat island article in Sustainability
This publication, Thermal Inequity in Richmond, VA: The Effect of an Unjust Evolution of the Urban Landscape on Urban Heat Islands, was an extension of my undergraduate research at the University of Richmond. Using previously gathered data on the urban heat island effect in Richmond, we analyzed the correlation between extreme heat and various urban and socioeconomic factors. I then contributed to the writing of the peer-reviewed version, which was lead authored by Kelly Saverino and published in February 2021 in the journal Sustainability.
Click figures to enlarge
The above table summarizes certain socioeconomic variables and the degrees to which they spatially correlate with the urban heat island effect. To calculate this, we organized existing data on air temperature by Census block in the city of Richmond along with socioeconomic data.
We then aggregated the distributions of these values across Census blocks, establishing the average values in the first and fourth quartiles, as well as the range in air temperature between each. Finally, we conducted t-tests between the distribution of each socioeconomic variable and the respective average temperature by Census block.
This yielded a wide range of results in terms of the relationships between extreme heat events and socioeconomic factors. Variables are sorted from negative (top) to positive (bottom) regarding how strongly they correlate with average air temperature. Factors such as household income, education level, and percent white population exhibited a fairly strong negative correlation with air temperature, while the proportion of the black population by Census block was positively correlated with prevalence of extreme heat events.
We also analyzed the relationship between air temperature and land cover factors, such as impervious surface and tree canopy cover. Finally, later analyses for this publication considered the relationship to HOLC ratings - the four-tiered categorization system upon which historical red-lining practices were based - which became a major theme in the article. The results for these subsequent analyses are in the second figure.
Among the more expected but still useful results were the strong correlations between extreme heat and lack of both pervious surface (i.e., intensely developed areas) and canopy cover. Additionally, it was found that extreme heat strongly correlates with the historic distribution of heavily red-lined areas, indicated as those with a HOLC rating of C or D.
Note: Kelly Saverino and Rong Bao created the above figures for this article. I contributed to the underlying matrix analyses, the early versions of which are described further under the 2019 section. The Cartography & Geospatial Analysis page contains more of my own work in this area.
The full Sustainability article with additional background and figures can be found here.
2020
Selected results from my December 2020 Sustainability article on urban environmental justice disparities in Richmond
This article, Assessing Inequitable Urban Heat Islands and Air Pollution Disparities with Low-Cost Sensors in Richmond, Virginia, was the main product of my undergraduate research at the University of Richmond. Advised by Dr. Todd Lookingbill, I collaborated with students and researchers at UR, Virginia Commonwealth University, and the Science Museum of Virginia to deploy a network of air quality sensors throughout Richmond. Upon identifying eight sensors with long-term, consistent data, I analyzed spatial and temporal trends in particulate matter (PM2.5) and temperature readings to determine possible underlying reasons for significant patterns.
This figure shows the variance in temperature readings from each deployed sensor. All conducted in R, this box plot is derived from an analysis of variance test on hourly average values during the ten hottest days of 2019 at each site. These distributions were run through a Tukey’s range test to determine which were significantly different from each other on a 95% confidence interval, projected onto a box plot, and colorized by category of significant difference. This figure is also represented in map form on the Cartography & Geospatial Analysis page.
The same process was used to create a box plot showing distribution of PM2.5 readings between locations, which yielded similar results but with less variability. Additionally, these findings undergirded the above 2021 publication in Sustainability written by Kelly Saverino, which was dedicated more heavily to studying the urban heat island effect and its ties to historic red-lining.
This graph represents a temporal component to compare trends in air quality between different locations on the ten hottest days of 2019. This perspective shows the difference in magnitude between PM2.5 readings and patterns that may emerge throughout the day in extreme heat scenarios. While inter-sensor disparities were present year-round, they were most prominent on hot days.
Click figures to enlarge
This figure plots average daily particulate matter (PM2.5) readings against temperature averages from mid-February to mid-November 2019. The overall trend exhibited a weak positive correlation, although a distinct cluster of high PM2.5 values emerged on the hottest days of the year (shown in red). Meanwhile, PM2.5 readings were highly scattered on cooler days, with no discernible correlation to temperature.
The full Sustainability article with additional background and figures can be found here.
2019
Foundations of my undergraduate research on urban environmental issues
The bulk of the project planning and analysis were conducted during my last three semesters of college, including full-time funded research during the summer before my senior year. In collaboration with other students at UR, we analyzed existing data on extreme heat throughout the city of Richmond relative to socioeconomic and geophysical factors, which were initially compiled in previous research by colleagues at the Science Museum of Virginia. Additionally, we built out a fleet of air quality sensors throughout the city, tested the reliability of sensors on spatial and temporal scales, and developed increasingly advanced methods for analyzing output data.
This figure summarizes a matrix analysis based on average temperatures at different times of day group by Census block in Richmond, which were measured in previous research by colleagues at SMV. These were each cross-referenced with socioeconomic factors such as income, racial demographics, and housing types, as well as geophysical variables related to urban development such as canopy cover and impervious surface.
As further described in the 2021 section on the resulting publication on the urban heat island effect, the distributions of comparable variables were analyzed via t-tests to determine the strength of their relationships and whether each correlation is positive or negative. In addition to subsequent analyses that included historic red-lining trends, this analysis was highly informative for the remainder of our team’s research in this area.
Click figures to enlarge
This graph was an early mockup used to visualize the spatial and temporal trends in PM2.5 readings from the eight sensors analyzed. Using daily PM2.5 averages during a sample week in July 2019, this provided an initial look at the degree to which air quality can fluctuate day to day. Similarly, it also showed how readings at each location will generally track with each other but will still exhibit a noteworthy degree of inter-sensor spatial variability.
The publications that stemmed from this work are linked in the above 2020 and 2021 sections.